The Dark Side of AI: Addressing Algorithmic Bias
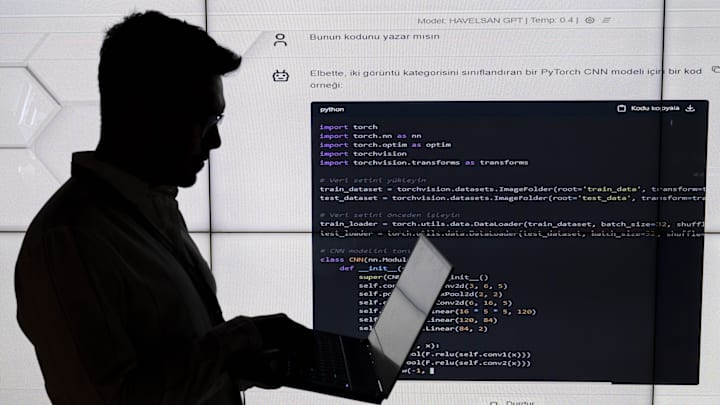
Artificial Intelligence has been hailed as the future of efficiency, innovation, and problem-solving. From helping us find the best route home to detecting medical conditions earlier, AI has undoubtedly improved our lives. But AI is far from perfect. Hidden beneath its polished exterior lies one of its biggest flaws: algorithmic bias. It’s a problem that doesn’t just impact data; it impacts people—often the most vulnerable among us.
Let’s dive into the “dark side” of AI and explore what’s really going on here.
What Exactly is Algorithmic Bias?
Algorithmic bias happens when an AI system produces unfair, inaccurate, or discriminatory outcomes. It’s not because machines are “bad” or malicious—AI doesn’t have intent. Instead, bias creeps in through the data it’s fed and the way it’s designed.
Think of AI like a student. If you give it biased textbooks, it’ll learn biased lessons. If historical data shows a trend of discrimination, the AI assumes that’s normal and reflects it in its decisions. And because AI systems are trusted to make “objective” choices, these biases can slip by unnoticed—until real harm is done.
Bias in Real Life: Stories That Matter
Unfortunately, algorithmic bias isn’t just theoretical—it’s happening right now. Hiring tools, for example, have been shown to prefer male candidates over female ones because they were trained on historical hiring data dominated by men. In a now-infamous case, an AI recruiting tool penalized resumes containing the word “women’s,” such as “women’s chess club,” reinforcing gender inequity.
Similarly, AI in the criminal justice system has caused widespread concern. Risk-assessment tools, used to predict whether someone will re-offend, have been criticized for being biased against Black defendants. These systems sometimes rely on skewed arrest data, which reflects systemic racism in law enforcement. As a result, individuals are unfairly labeled as “high risk,” impacting their sentencing and parole opportunities.
Even healthcare hasn’t been spared. AI tools designed to diagnose diseases or prioritize patients for treatment sometimes fail to account for underrepresented groups. For instance, systems trained mostly on white patients have misdiagnosed conditions in Black or brown individuals, creating dangerous gaps in care.
How Does This Happen?
Bias in AI usually comes down to a few root causes:
- Skewed Data: AI is only as good as the data it’s trained on. If that data reflects existing societal inequalities—like wage gaps or systemic racism—AI learns to replicate and amplify them.
- Lack of Diversity in Design: The teams building AI systems often lack diversity themselves. When designers and engineers come from similar backgrounds, they may overlook biases or fail to recognize how AI could impact marginalized groups.
- Oversimplified Models: AI systems often reduce complex problems to patterns or numbers, ignoring context. Real-world factors like socioeconomic status, historical discrimination, and lived experiences can’t always be boiled down into clean datasets.
Why Should We Care?
Bias in AI isn’t just about bad code—it’s about real people being denied opportunities, facing unfair treatment, and perpetuating the inequalities that already exist in society. AI systems are used to make critical decisions about hiring, education, healthcare, loans, and even law enforcement. When bias goes unchecked, it reinforces injustice on a larger scale, often impacting those who already face systemic barriers.
Worse yet, because AI systems are seen as “neutral” or “objective,” their decisions carry a weight that human decisions often don’t. It’s harder to argue with an algorithm than with a person.
Addressing the Problem: What Can We Do?
The good news? Bias in AI can be addressed—but it’s going to take intentional effort.
- Diversify the Teams Behind AI: The people building AI systems must reflect the diversity of the world we live in. Different perspectives can help spot biases that might otherwise go unnoticed.
- Audit and Test for Bias: Regularly reviewing and testing AI systems for bias can help identify problems early. Just like you’d debug code, we need to debug bias.
- Improve Data Quality: Feeding AI more representative, balanced, and inclusive data can reduce bias. This includes considering how historical injustices influence data and accounting for those gaps.
- Increase Transparency: Companies and developers should be clear about how their AI systems are built, what data they use, and what limitations exist. Transparency builds trust and allows for accountability.
- Regulation and Policy: Governments must step in to set standards for fairness in AI systems. Ethical guidelines and regulations can help ensure companies prioritize fairness over speed or profit.
The Path Forward
AI is here to stay, and that’s not a bad thing. When done right, AI can transform industries and improve lives. But for AI to truly serve humanity, we must address its biases head-on. It’s not enough to accept these systems as they are. We need to question, challenge, and refine them to make them fairer, smarter, and more ethical.
The next time we marvel at what AI can do, let’s also ask: Who does this system help? Who does it harm? And how can we make it better? Because at the end of the day, technology is a tool—and we decide how it’s used.